What are the potential applications, benefits and risks of AI for dementia researchers, patients, and research itself? What is the most optimistic scenario for how things could turn out if we employ AI in dementia research, and how do we work towards this end, while mitigating any potential harms. These are questions I’ve been trying to address in a series of now three blogs. In a first blog, I discussed how individuals can use AI in personal, day-to-day research tasks. In a second, inspired by a debate at the 2024 ARUK Conference, I discussed the opportunities for using AI in dementia diagnosis, trials, and healthcare, as well as the potential harms. The question of the debate was actually much wider though, asking what benefits AI could bring to dementia research at as whole. So, in this blog, what I want to discuss is everything else: how can AI assist us at the ‘basic science’ level of dementia research?
You might imagine that this is going to mostly talk about AI designed drug discovery and big data and other such topics, and we will get there, but there are so many more opportunities here, so we are going to begin with some ideas that I think are direly underdiscussed, underutilised, and theoretically already possible.
-
Literature Searches
When I explain how fundamental scientific research works – the whole ecosystem of fundamental discovery to experiments to writing papers to publication – one question my dad has routinely asked is “OK – but who is sorting through and reading all the papers at the end? Who is collecting all the discoveries and working out what is important, what is not, what ideas are worth pursuing? Who is putting ideas from different labs, different countries together, to come up with new directions?”
In some senses, it’s everyone. We all read the literature, present at conferences, hear ideas, and are inspired by these interactions. We write literature reviews on areas of our own expertise, combing through papers to come up with summaries and insights for everyone else. Pharmaceutical companies search through conferences and journals for promising new targets to follow up on. Journals and reviewers make decisions about which papers are most important and relevant, and those judged to be more impactful get sorted into the most viewed journals. In this sense, science works as a live information engine, a highly distributed machine working collectively across all its constituent members.
In another sense though, it’s also no one. No one has read all the papers. No one actually has time to properly read much literature at all. Even if you read 100-200 papers a year, which would be a truly absurd amount, it would pale in comparison to the 1000s of articles published on dementia research every week. Sure, not all of it is relevant, but even small subfields still have insurmountable and growing avalanches of information that no one human can consume, interpret, and integrate. Furthermore, if you limit yourself to reading only those articles relevant to your own work, you miss all the ideas from adjacent fields, or even completely different fields, that might nevertheless apply and give you a breakthrough. Reading articles or going to talks at random is just that – random. Not particularly efficient, and not necessarily effective. Putting together literature reviews, especially meta-analyses and systematic reviews takes a huge amount of time and effort, even when they’re non exhaustive. Moreover, many of us are limited to reading the literature in one or a couple of languages. There is simply too much information to sift through, and no time or physical mental capacity to keep up or process it, let alone find all the things that are even relevant to you.
Here is where AI comes in. We should be able to have AI language models trained and constantly updated on the entire of the dementia research literature – and neuroscientific and scientific literature beyond that. These should be able to answer questions about directions the field is heading in, summarise latest findings, and find patterns in data. But this is just the basics. AI models should be able to suggest the best and most relevant papers that might have ideas, data, or methods I’m interested in. AI should be able to read all of my papers and ideas and then tell me who in the world is doing similar research to me, who is doing complementary work, who would be best to collaborate with, and who is probably already about the publish the project I’m starting to work on. If I’m stuck on a research question, an AI that’s read all of the scientific literature ever produced should be able to tell me who would be best placed to answer it – even and especially if they’re not from the dementia research field themselves.
It doesn’t have to be big picture theoretical questions either. Imagine I want to use a commonly used drug like rapamycin in my cell model, to test the function of a process like autophagy, but I don’t know what concentration I should use. AI, having read all the papers, should be able to give me a list of all the papers that have used rapamycin in cell models, what concentration they used, what cell models they used, and what the effect was. Same if I want to try using a new antibody that I haven’t tried before. My life would be so much easier if I could ask an AI to list all the times a particular antibody was used, what concentration it was used at, what kind of sample it was in, and show me what their images looked like.
But what if I’m interested in a particular topic or concept – for me personally, it might be selective vulnerability. Or it might be differential vulnerability. Or it might be selective neuronal vulnerability. Or specific neuronal vulnerability. Or regional vulnerability. Or whatever key words the authors chose to use. Should I search for induced pluripotent stem cells? iPSCs? iPS cells? hiPSCs? How about if I want to search for papers on autophagy? Or macroautophagy? Or lysosomes? Lysosome? Endolysosome? Lysosomal function? Endolysosomal Network? [Can you tell this has frustrated me before?] The point here is that trying to search for terms without standardised terminology is a pain in the neck, but AI-powered natural language models should be able to perfectly interpret what I am interested in, based on my question but also what it knows broadly about my interests and search history, and then go and find everything relevant to that, regardless of the specific terminology or exact keywords used.
What about when we do a literature search for papers on a particular topic – and the search returns 15 pages of results. Are you going to read every title? Every abstract? Will you click all the way to page 15? What if the answer you were looking for was in a paper on page 11, which you had glazed over while you scrolled through? So much literature, that may be useful, has likely been missed or overlooked over the years. Similarly, it may be easy to think something is a novel idea, worth pursuing, when in fact it has been examined before, and you just didn’t come across that particular paper, Worse, it already be underinvestigation by another lab, and you didn’t happen to be at the particular conference session where they presented the progress they’d made so far. AI, that has read all literature, conference abstracts, departmental profiles, lab websites, project listings, grant approvals, etc., can help on this front.
AI has issues that need to be recognised here, when it comes to hallucinations – i.e. spontaneously coming up with information that has no basis in fact, but sounds plausible. This issue does somewhat undercut the potential use for AI in summarising information from papers or producing literature reviews. However, designers of these large language models are already finding ways to mitigate these. Getting AI models to provide links to sources, or search for exact quotes is one way of reducing this issue, for example. This also makes them readily fact checkable. Moreover, as AI models get better, and are trained on more data, error rates have been decreasing, with GPT4 producing a 3-5% error rate, and models improving on even this since.
The use of AI here isn’t to provide a perfectly accurate summary of content. That is one application, but really what we want it to do here is prioritise and filter the vast world of scientific content for us – to provide starting points for us to spring off. To be the one person, as my Dad might imagine it, who has read and interpreted all the literature, made all the connections, and can point them out to people as needed or relevant.
-
Enhanced Conferences and Collaboration
As alluded to earlier, another key application of an AI language model that has read all of the literature should be to circumvent the randomness of collaborations and conference meet ups. We’ve all heard the idea that when you go to conferences, sure, you’re ostensibly there for the talks and posters, but really, it’s the watercooler chats and people you bump into in the corridor outside – that’s where the real action happens, where collaborations spark, and chance meetings burgeon into new discoveries.
This view of conferences is absurdly romanticised, and inefficient. If I’m going to a conference, I want an AI model to have read my abstract, and to have read my bio, as well as the abstracts and bios of everyone else there, and then suggest to me whom I should talk to. I want to be able to tell an AI Chatbot what questions I’m interested in, and have it tell me who is coming to this conference that can help me. Maybe these things are easier at smaller meetings, but especially for big conferences with thousands of people like ADPD, FENS, AAIC, SFN, etc., this would be invaluable. I cannot possibly read through all 1500 abstracts for ADPD, and even searching through all my key terms in the abstracts is a day’s worth of work, let alone all the talks. Surely an AI that understands what I am interested can suggest what talks I might want to go to, and then, having looked at both of our conference programmes, suggest times to go and meet people that might be good to collaborate with when we are both free.

AI is a growing area of dementia research. It holds huge potential for more accurate, early diagnosis of the condition as well as predicting its progression.
If we want, we should be able to have AI point out who at a conference we’ve talked to before at at previous meetings. It should be able to know which papers we’ve read, and point out authors in attendance whom we might want to talk to. It could even be aware of whom we follow on social media, whose posts we interact with, whose work we admire, and whom we might want to meet in person – and prompt us to reach out when we’re attending the same meeting. Networking help!
And yes, these ideas are inspired by all the times I’ve been at conferences and realised only too late that the person speaking wrote one of my favourite papers or the times I have glanced at someone’s name tag as they walk past, only to realise I really wanted to talk to them, or the times that I missed or overlooked a talk by someone that in retrospect, would have been really interesting. But it’s also inspired by all the times that I’ve found myself in a random talk or in front of a random poster, not expecting it to be particularly interesting or relevant, only to find it absolutely fascinating and important to my project, or using a method I could apply to my own experiments. We shouldn’t be relying on random chance for these things to happen!
Excitingly, conferences like BNA International Festival have already begun trying AI-based recommendations of people to contact and sessions to attend based on keywords and submitted abstracts. While not great in its first iteration, with limited data to work with, the premise has huge potential. To be clear, the idea here is not simply an AI gimmick for conferences. The hope is that AI can help better connect scientists from all around the world, and enable us to stop relying on random chance and produce more fruitful collaborations.
The current scientific paradigm has scientists in an uneasy middle ground between wanting to reach out and collaborate on the one hand, and competing to publish new discoveries first, holding methods and data secret until then. AI could change all of this, with an ability to predict who is best suited to working on particular questions or who may already be working on or about to publish particular projects, based on their previous papers, funding applications, hiring actions, conference presentations, equipment purchases, and existing collaborations.
This could also make finding new labs and projects vastly easier for students and postdocs in search of projects and jobs, or a home for their ideas. Instead of trawling through department faculty profiles, relying on word of mouth, or hoping to randomly bump into someone at a conference, AI should be able to tell us, based on a brief project outline, whose lab has the funding, equipment, and expertise necessary to house such an idea, and who else might be able to collaborate. If a lab is looking to hire someone with a particular skillset, AI should be able to tell them who at a conference would be a good fit. The potential for AI to transform science from a highly competitive landscape, where information is hoarded and multiple scientists race in parallel on similar ideas, to a more collaborative one where scientists all over the world become connected in a more targeted and transparent manner, is huge. Moreover, the potential for AI to transform collaboration and discovery from a semi random exercise that largely benefits those with the funding and availability to go to conferences and proactively reach out to people, to a more targeted and equitable system, is also great, and I am excited for the benefits this will bring to research.
-
Analysis of Big Data
The above applications of AI are more on the meta-science front, or how the process of research works, and how it might be improved. But what about experimentation and data analysis itself? Here too, AI is already becoming increasingly important and useful. Datasets are ballooning. Studies now involve more patients, more cells, more genes and proteins, more longitudinal timepoints, more electrodes, more images in high throughput screens, and higher density readings from wearable devices.
This is, largely, a good thing. The brain is the most complex thing in the known universe, and these are among the most complicated diseases confronted by medical science. We need high levels of granular data across large populations to inform our understanding of what are highly heterogeneous conditions. Using AI to analyse these datasets isn’t just useful, it’s necessary. Moreover, AI can both interrogate individual datasets, and find subtle patterns across multiple modalities. Consider the case of mixed pathologies, as described in a previous blog – there are many dementia syndromes, driven by many different diseases, featuring many different combinations of many different pathological changes. These pathologies, be they protein accumulations, vascular lesions, metal ion deposits, synaptic loss, inflammation, glial scarring, or neurodegeneration, may manifest in different brain regions or different cell types, and this all likely informs the clinical presentation and progression of disease, as well as the patient’s response to different therapeutics. These are all likely driven by a patient’s genetics, their lifetime exposures (e.g. to stress, air pollution, infections, or other environmental toxins), and their behaviours (such as exercise, diet, education, sociality, etc.), and the biophysics of the pathologies in question. There are patterns to be found amid all these datapoints, and a huge opportunity to have a more personalised understanding of what causes dementia in different people, though not one that humans can achieve alone, without AI to sort through all this data.
AI excels at quickly finding subtle changes and patterns in large volumes of data that we might not even think to look for. Integrating AI into analysis has enabled us to vastly increase the power of our studies. Compare the paradigm of neuropathological examinations in the past, where a vast team of researchers would have had to manually count stained cells, and physically measure neurites if we wanted to examine patterns of neurodegeneration. This was both enormously time consuming and expensive. Now, this process of image analysis that would have taken multiple people days to weeks can be done by one person, and an AI, in minutes, enabling vastly more samples to be processed in far shorter a time. Some might be concerned about AI automation replacing the jobs of scientists, but I would argue there are better things for researchers to be doing than counting cells across hundreds of pictures.
Moreover, AI-driven automated image analysis can examine not just number of cells and neurite length, but all kinds of image features from change in neurite branching and thickness to other architectural changes we might not have considered, identifying changes and patterns that would have been missed otherwise. Applications like cell painting, where various organelles and other structures of cells are all stained in different colours, allowing AI algorithms to examine hundreds of features of the cell at once and identify differences under various conditions, are enormously powerful for taking an unbiased approach to understanding the complex fundamental biology of these diseases.
Consider also techniques involving the recording live brain cell activity. Understanding neuronal firing patterns is crucial to the fundamental functioning of the brain, and is one of the changes that is thought to first occur in dementia. Previously, this might have involved sticking a needle into individual cells and performing slow, painstaking recordings in low powered experiments, measuring 10s of the possible millions of cells. Alternatively, it may have involved recording from scalp electrodes on a patient, reading out brain waves using EEG, and hoping to see dramatic changes. Now, using technologies like multielectrode arrays, Neuropixels probes, electrocorticograms that record from thousands of tiny electrodes inside the skull, and EEG arrays with as many as 256 electrodes sitting outside the head, we can collect enormous amounts of data about the functioning of many cells at the same time. This kind of signalling information, however, is unfathomably complex. The data would be largely useless, without AI to analyse it. Maybe we could identify big trends by eye – but could you really be confident that you could find all the patterns amid 256 separate electrodes recording brain activity? Could you really tell the difference between the firing patterns of a collection of hundreds of neurons firing in a disease versus healthy state? AI enables us to find the earliest, the most subtle, and most obscure changes that may pertain to disease. It’s capable of sifting for patterns in firing rates, amplitude changes, inter spike intervals, synchronicity, changes in oscillation frequency and power spectra, and all kinds of other features that are beyond human capacity.
Another interesting example is in speech changes. There is increasing evidence that speech can be a highly sensitive biomarker for a myriad of diseases or other heath states, from colds and flus to mental health conditions, pregnancy, and of course, dementia or movement disorders (and not just aphasias). Changes could be in the sound of speech, the speed and cadence at which a person talks, their pronunciation of certain words or sounds, and even changes in sentence length, vocabulary, and fluency. This is a perfect use case for AI – interpreting different modalities of data (sound, natural language processing), analysing huge amounts of longitudinal data and searching for subtle patterns and changes occurring over years that may not be obvious to a clinician in a 5-minute observation. AI would then be able to tie back different patterns of changes to different diseases, enabling us to develop new, non-invasive, passive, sensitive, and potentially highly specific biomarkers.
To be explicit: analysing this kind and scale of data – be it genetic, structural, electrical, sonic, linguistic, or anything else, would be impossible without AI. These algorithms enable a huge scope of new research, better powered, at large scale, for lower cost, which could greatly increase our understanding of complex disease biology, and the speed at which we do so.
-
AI Simulation and Modelling
Separate to the analysis of large datasets is what I have broadly categorised as simulation and modelling. This goes one step further from pattern detection amid large datasets, and starts to make predictions, another strength of AI. This has all manner of applications, the most obvious of which might be AlphaFold. This AI programme, built by Google’s DeepMind team, is trained on all the known protein sequences and structures to predict structures of proteins that we don’t yet know the structure of, and to predict how protein structures might change given certain mutations or conditions. Experimentally determining protein structure is extremely difficult and time consuming. AlphaFold, in predicting the structure of all known proteins, has completely changed the field. Understanding the three-dimensional structures of proteins is critical for deciphering their functions, interactions, and role in disease pathology, especially given that the hallmark of neurodegenerative diseases is the misfolding and aggregation of various proteins.
Moreover, understanding the structure of proteins, not just those directly affected by disease, but also those involved in disease related pathways, enables us, and by us, I mean AI, to identify target sites for drugs, antibodies, or other means of intervention. AI simulation and modelling techniques can accelerate the drug discovery process by predicting the binding affinity and efficacy of simulated drug candidates targeting specific proteins involved in dementia. Using computational methods such as molecular dynamics simulations and virtual screening, researchers can identify promising drug candidates, optimise their chemical structures, and predict their pharmacokinetic properties, all before conducting costly and time-consuming experimental studies.
This takes us to the potential for so called ‘in silico’ experiments. Rather than having to house animals and go through the ethics concerns of conducting experiments ‘in vivo’, and rather than having to go to the expense and effort of painstakingly growing cells for months to do experiments ‘in vitro’, some experiments can be simulated on a computer, ‘in silico’. This is still in relatively early days of development, but is already being applied to drug screening applications, where experiments can be conducted at vast scales much more quickly and cheaply, to identify top candidates to then validate in real world experiments. In the future, these AI-powered simulations may allow us to test hypotheses, simulate different biological processes, test the effects of genetic variations or environmental factors on cell function and disease progression, reduce our use of animal models, and more.
Conclusions:
This series was, in part, inspired by the ARUK Conference debate on whether AI could improve dementia research and patient outcomes. While the debate itself largely focused on potential patient facing applications in dementia diagnosis, as discussed in a separate blog, the reality is that many applications of AI are not in the future but already in use, and already benefiting dementia research. AI-driven analysis is already transforming how we understand disease, and enabling us to interrogate larger, more complex, and better powered datasets, in ways that would have been previously impossible. Its ability to predict and simulate based off vast reams of collected data is already being put to enormous effect, with huge potential for accelerating target discovery through more efficient and expansive literature searching ability, huge potential for drug discovery through molecular and target simulations, and huge potential to make the whole process much faster and cheaper through using in silico screening and filtering. This also has not been an exhaustive discussion – AI could also help dementia research by spotting scientific fraud more quickly. Data sleuths are great, but there are not enough to be able to cover all research at pace, and the field has already been marred by major fraud scandals. AI automation of basic research admin tasks could also free up time to do more actual science, which would not only be beneficial for the pace of science itself, but also the rate of people dropping out of research in frustration at the admin burden.
These uses are not without their risks and concerns – AI itself remains expensive, both financially and environmentally, though less and less so, and these, as well as privacy concerns, I have discussed elsewhere. Pitfalls in hallucinations should be taken seriously, though these are readily able to be overcome if AI is used to generate suggestions rather than summaries, and thus can easily be fact checked, and everything will need to be experimentally validated anyway.
Outside of the biology itself, the potential for AI to transform how the process of science, science communication, and scientific collaborations for the better is also immense. These in particular present minimal risk – most of the data these models would be trained on are public, so they don’t have the same privacy concerns that patient facing applications do.
Ultimately, this is largely why I actually believe that AI can be a game changer for dementia research, and the benefits outweigh the risks. Neurodegenerative diseases driving dementia are simply too complicated for our simpler tools. Patient conditions are too heterogeneous and mixed pathologies and their multifactorial aetiologies too varied, for us to take anything less than a big data approach. This requires AI, and in doing so, could allow us to personalise dementia care, identify disease far earlier than we currently can, and study the earliest stages of dysfunction that are presently inaccessible to us. These things might be possible without AI – but would take far longer, cost much more, and require a vastly bigger scientific workforce than we have.
Even if we decide that AI is too risky to be applied in patient facing settings such as diagnosis and clinical decision making, the potential for speeding up drug discovery and accelerating fundamental research through targeted collaborations and more efficient, comprehensive literature searching is immense. It would transform our engine of knowledge from one running on chance discovery to one designed to best harness and coordinate the collective intelligence of the scientific community. It represents a paradigm shift in how we generate scientific understanding of the complex fundamental biology of disease that can accelerate our journey to discoveries, ultimately improving outcomes for researchers and patients alike.
It is clear, therefore, what AI does and might be able to do for dementia research. What remains, then, is to make sure it happens in the way we want it – safely, responsibly, and ethically.
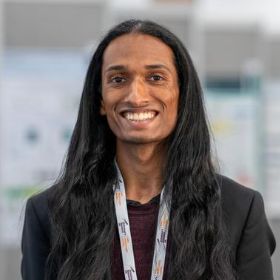
Ajantha Abey
Author
Ajantha Abey is a PhD student in the Kavli Institute at University of Oxford. He is interested in the cellular mechanisms of Alzheimer’s, Parkinson’s, and other diseases of the ageing brain. Previously, having previoulsy explored neuropathology in dogs with dementia and potential stem cell replacement therapies. He now uses induced pluripotent stem cell derived neurons to try and model selective neuronal vulnerability: the phenomenon where some cells die but others remain resilient to neurodegenerative diseases.